Sort/Filter Table Data¶
Sorting and filtering is a common way to manage and inspect table data. These operations are collectively called “proxy” because both of them intermediary map the original data to new indices.
Filtering¶
Use filter functions¶
You can set a function that maps a DataFrame
to a 1-D boolean array as a filter
function. This is a most straightforward way.
def filter_func(df):
return df["label"] == "A"
table.proxy.filter(filter_func)
# or equivalently, use decorator
@table.proxy.filter
def filter_func(df):
return df["label"] == "A"
This example is essentially equivalent to slicing a DataFrame
by df[df["label"] == "A"]
.
If the table is
A |
label |
|
---|---|---|
0 |
2 |
A |
1 |
3 |
B |
2 |
6 |
B |
3 |
4 |
A |
then it looks like following after applying the filter.
A |
label |
|
---|---|---|
0 |
2 |
A |
3 |
4 |
A |
Simple filtering in GUI¶
In right-click contextmenu, you can select will be anchored at the corner of the column section during
the table being filtered. You can update or remove the filter by clicking the button.
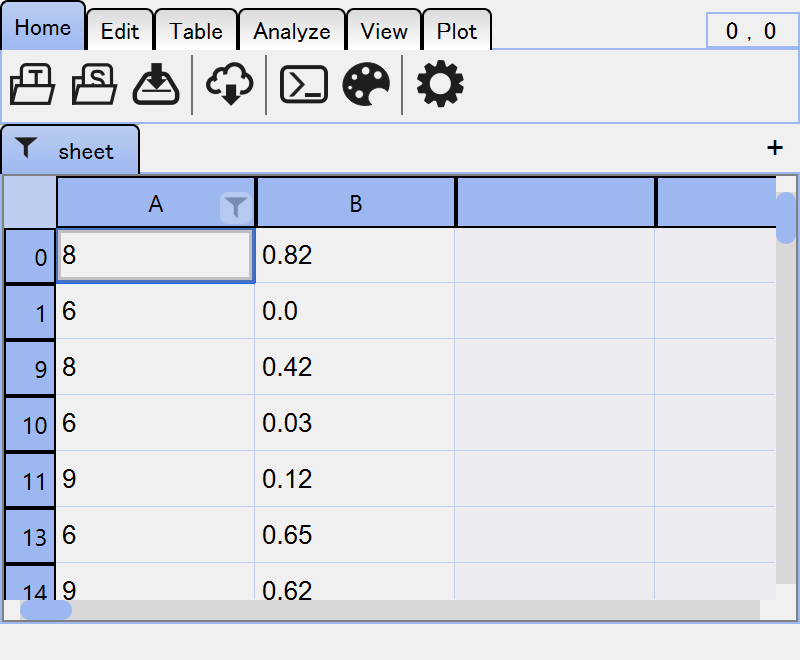
Use query-style expression¶
Instead of a function, you can also set a query-style expression as a filter.
table.proxy.filter("label == 'A'")
See the API reference of pandas.eval for details of the syntax.
If the expression can be interpreted as a simple column-wise filter, button will
similarly be added.
Query-style filtering in GUI¶
You can also open a overlay dialog to filter the table data from the button in
the toolbar.
The line edit for filter expression supports auto-completion (Tab) and history browsing (↑, ↓).
Sorting¶
Use sorting functions¶
Similar to filtering, you can also set a function for sorting. In this case, the function
should map a DataFrame
to a 1-D interger array, just like argsort()
.
def sort_func(df):
return df["x"].argsort()
table.proxy.sort(sort_func)
# or equivalently, use decorator
@table.proxy.sort
def sort_func(df):
return df["x"].argsort()
If the table is
x |
y |
|
---|---|---|
0 |
2 |
a0 |
1 |
3 |
a1 |
2 |
1 |
a2 |
3 |
0 |
a3 |
then it looks like following after sorting.
x |
y |
|
---|---|---|
3 |
0 |
a3 |
2 |
1 |
a2 |
0 |
2 |
a0 |
1 |
3 |
a1 |
Sorting function doesn’t always have to be surjective, i.e. it can return only a subset of the source indices.
@table.proxy.sort
def sort_func(df):
# return the top 10 rows
return df["x"].argsort()[:10]
Sort by a column¶
In most cases, you’d like to sort a table by a column, in ascending or descending order.
The sort()
method also supports this use case, by passing by
argument.
table.proxy.sort(by="x") # ascending order by default
table.proxy.sort(by="x", ascending=False) # descending order
Multi-column sorting is also supported.
table.proxy.sort(by=["x", "y"])
Sort in GUI¶
You can sort selected column(s) by clicking button in the toolbar.
Edit Cells during Proxy¶
You can edit cells while the table is sorted/filtered.
Suppose you have a table like below
A |
B |
---|---|
0 |
row-0 |
1 |
row-1 |
2 |
row-2 |
3 |
row-3 |
4 |
row-4 |
5 |
row-5 |
6 |
row-6 |
7 |
row-7 |
8 |
row-8 |
9 |
row-9 |
and applied a filter by
viewer.current_table.proxy.filter("A % 2 == 1")
A |
B |
---|---|
1 |
row-1 |
3 |
row-3 |
5 |
row-5 |
7 |
row-7 |
9 |
row-9 |
Here, you can edit, or paste data directly (The edited cells are highlighted in red).
A |
B |
---|---|
1 |
row-1 |
3 |
X-3 |
5 |
X-5 |
7 |
X-7 |
9 |
row-9 |
After removing filter (viewer.current_table.proxy.reset()
), you’ll see the cells
are properly edited.
A |
B |
---|---|
0 |
row-0 |
1 |
row-1 |
2 |
row-2 |
3 |
X-3 |
4 |
row-4 |
5 |
X-5 |
6 |
row-6 |
7 |
X-7 |
8 |
row-8 |
9 |
row-9 |
Column Proxy¶
New in version 0.5.5.
Another way to sort/filter table data is the column proxy.
def filter_func(s: str):
return "_mean" in s
table.columns.filter(filter_func)
# or equivalently, use decorator
@table.columns.filter
def filter_func(s: str):
return "_mean" in s
table.columns.sort(ascending=True)